What Is Decentralized Artificial Intelligence? A Beginner's Guide
March 11, 2024
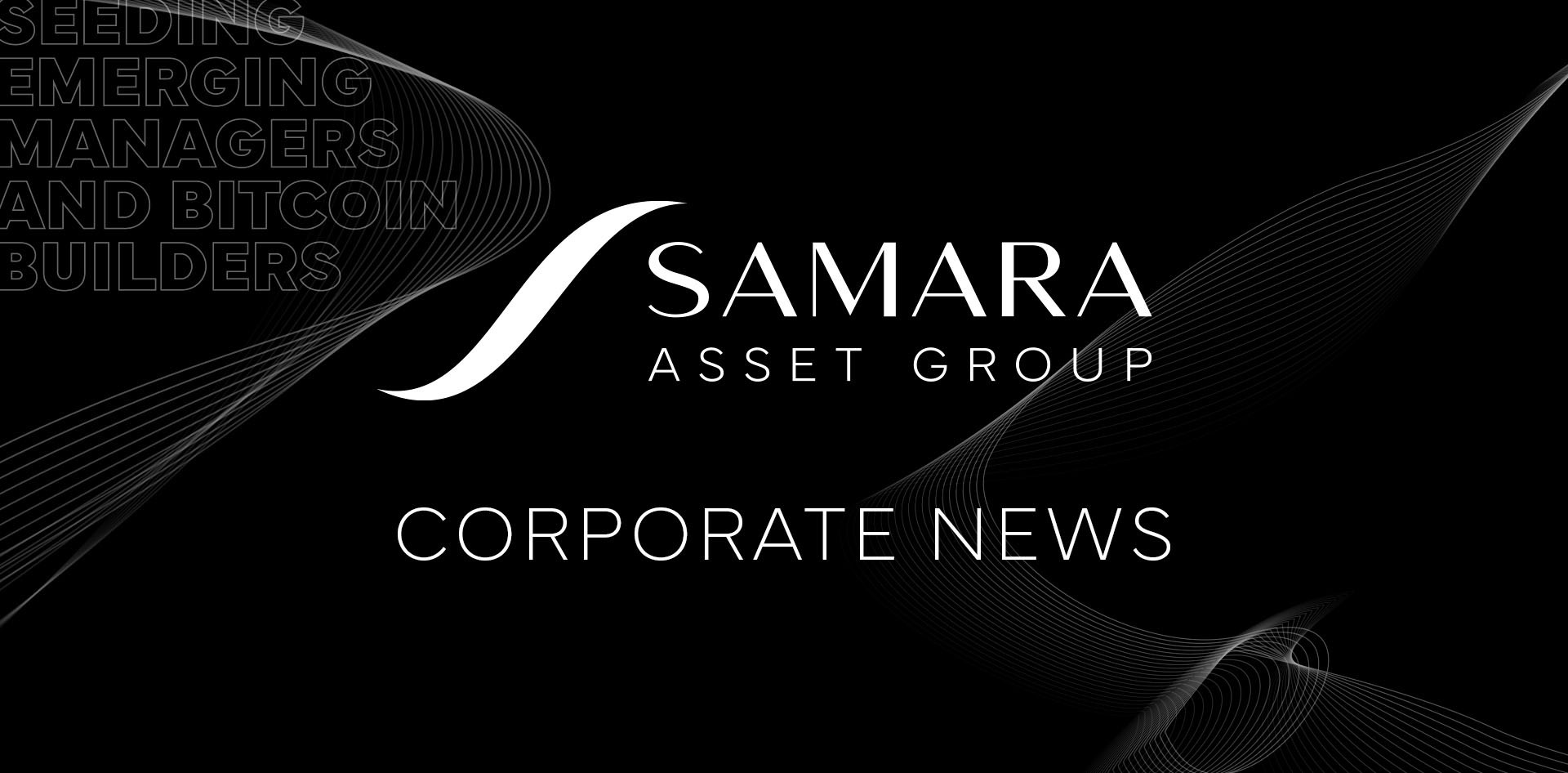
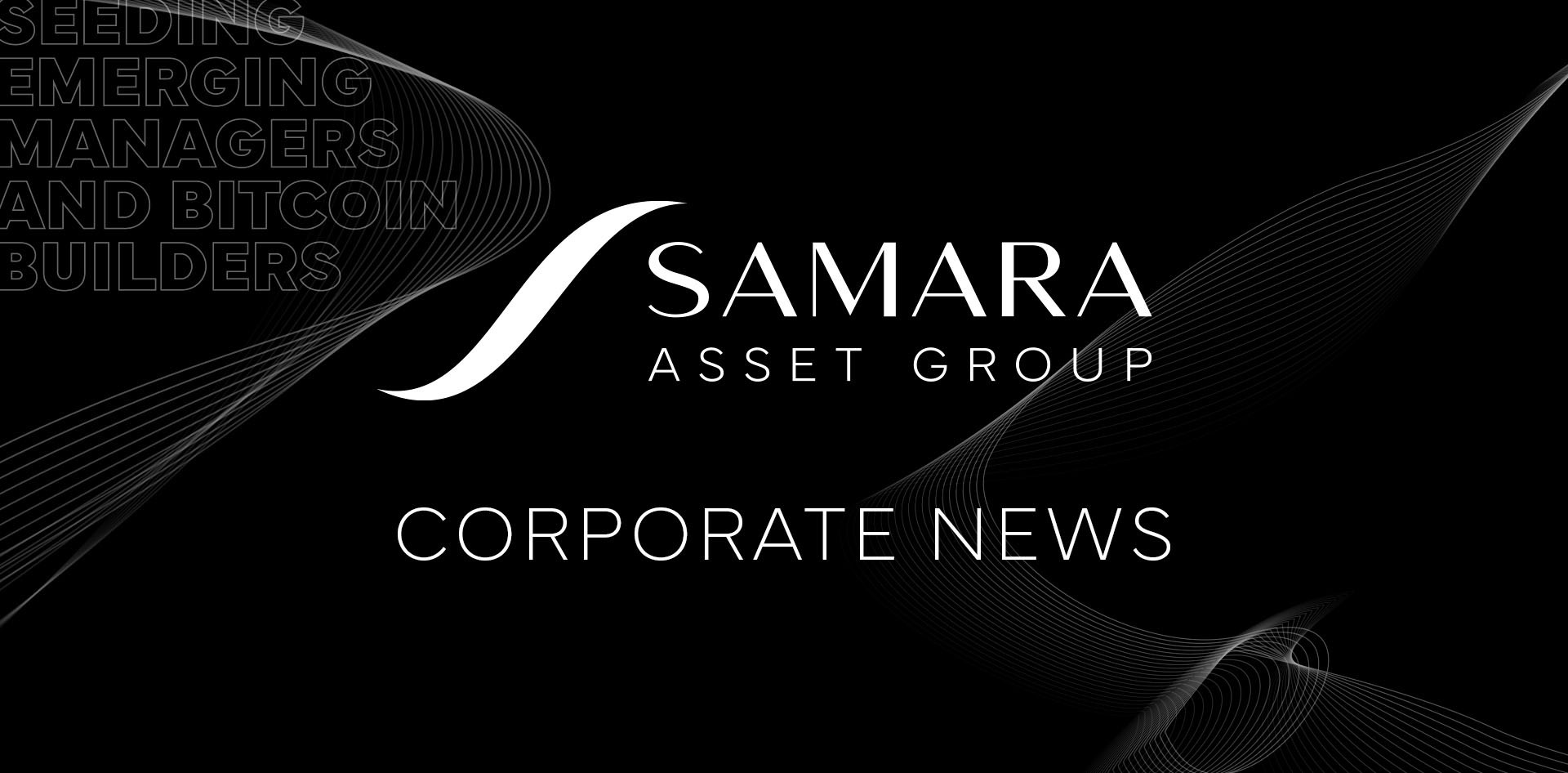

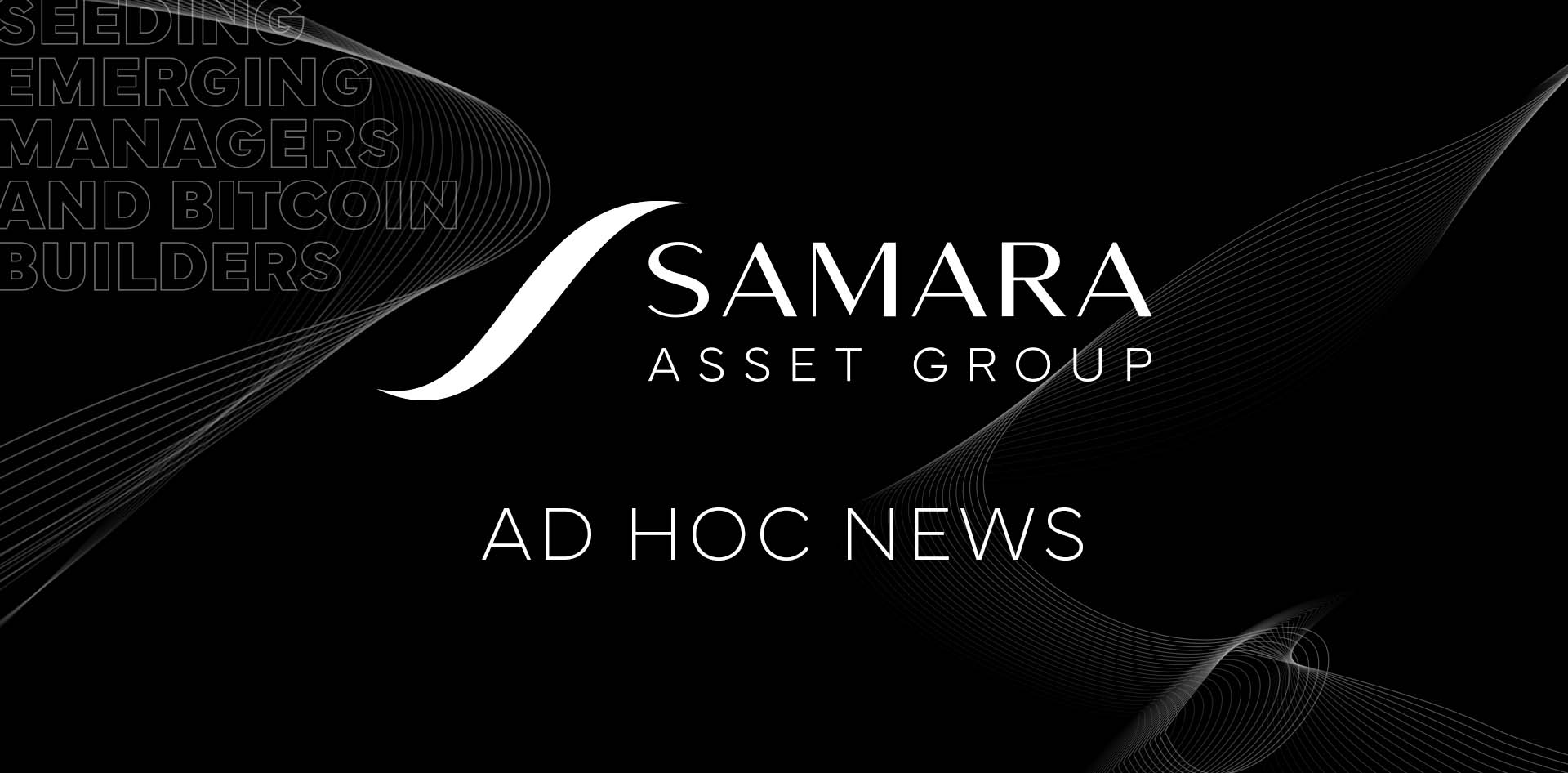
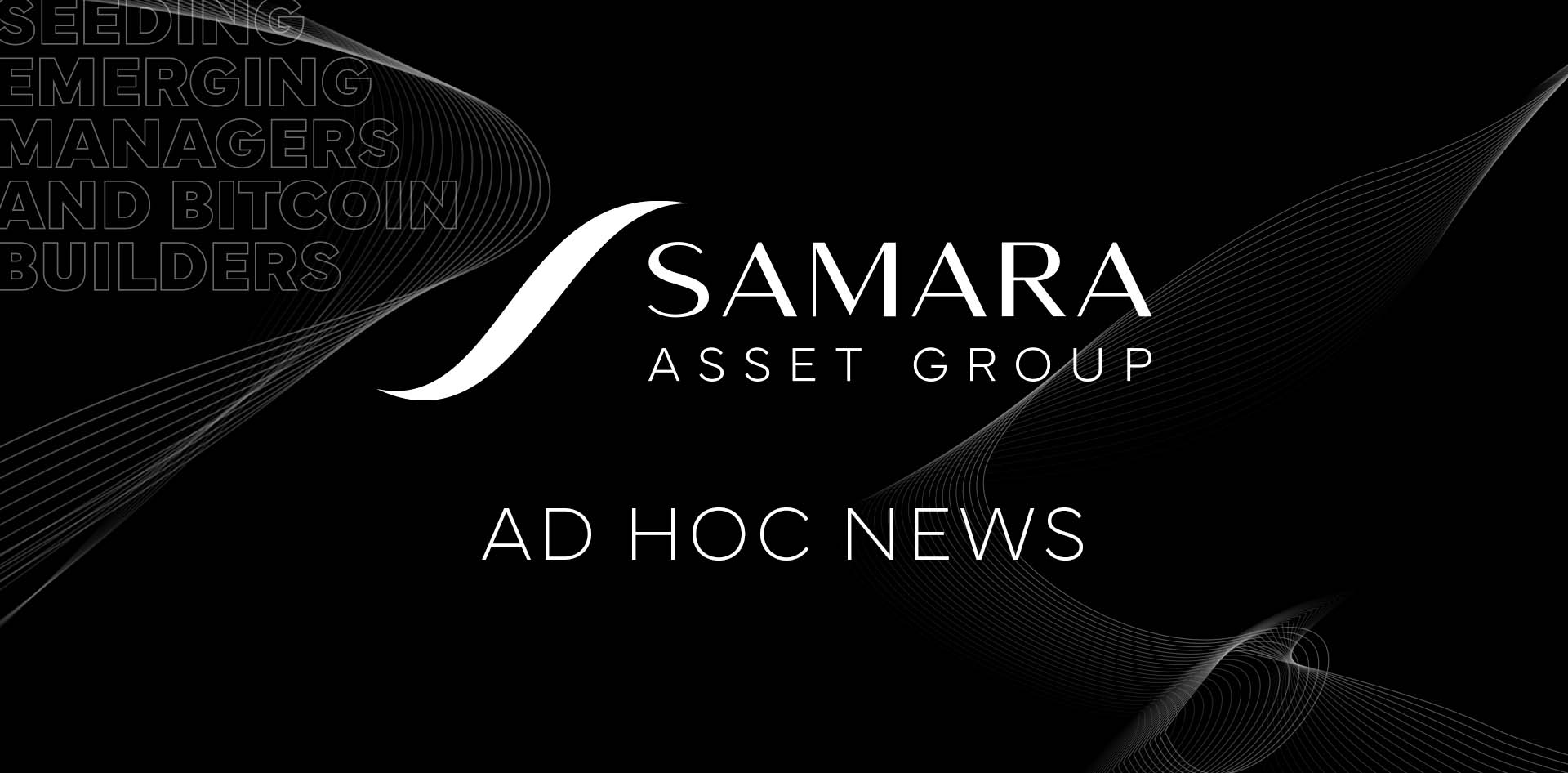
Decentralized Artificial Intelligence (DAI) integrates blockchain technology with AI to enhance machine learning capabilities, promising to restructure the future of intelligence. This guide explores DAI's core principles, delving into its potential benefits and practical applications.
Table of Contents
What Is Decentralized Artificial Intelligence?
DAI refers to a decentralized artificial intelligence system that uses blockchain technology to process, distribute, and store data across a network of nodes.
Unlike regular AI systems, the decision-making process in the DAI systems is decentralized, meaning it's based on consensus across a global network instead of being controlled by a singular central authority.
The element of decentralization ensures the potential of AI isn't hoarded by a few individuals, making it a more transparent, secure, and reliable approach to AI functioning.
Decentralized artificial intelligence increases the accessibility of AI and democratizes its use by making it more widely available and adaptable.
DAI has applications across multiple industries, including healthcare, finance, and energy, where enhanced transparency, security, and efficiency are paramount. Some core elements of these systems include decentralized applications (DApps), federated learning, smart contracts, and encryption technologies.
How Does Decentralized AI Work?
The decentralized AI setup involves different nodes working together to contribute their computational resources by providing data and processing power to collectively train models and make predictions.
Federated learning is a critical element of DAI, allowing the collaborative training of AI models across a network of decentralized devices that don't share raw data. Each device processes its raw data individually, updates models, and then shares these updates with a central server. This process enhances data security and minimizes privacy concerns.
DAI systems rely on interconnected devices (nodes) that run their own AI algorithms locally. The devices can be computers, smartphones, Internet of Things (IoT) devices, or special AI hardware. These nodes communicate within themselves to facilitate AI inference and collaborative learning across the network.
Where traditional AI systems depend on a centralized server for decision-making, DAI decentralizes decision-making by enabling AI models to use cutting-edge devices to make decisions and predictions.
Benefits and Drawbacks of Decentralized AI
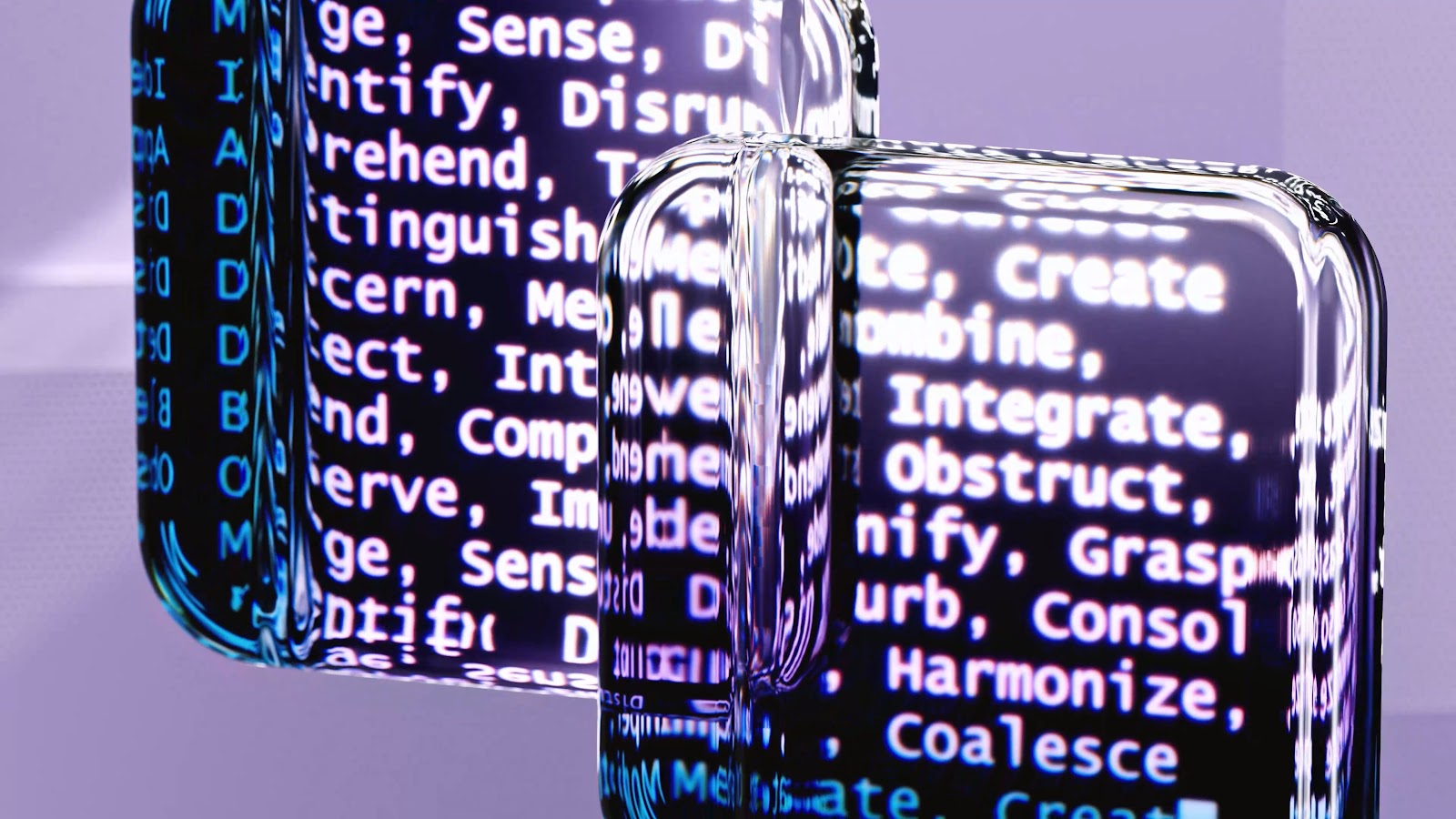
Decentralized artificial intelligence brings a host of benefits as well as challenges. Let’s have a look at them.
Pros
- Increased privacy and security: DAI mitigates the risks associated with cyberattacks and data breaches by eliminating the need to send sensitive data to external servers.
- Enhanced speed: Edge devices in decentralized AI ensure data is processed locally, reducing latency and prompting real-time decision-making.
- Efficiency and reliability: DAI provides excellent reliability in critical applications as it can continue serving efficiently even if a few nodes or devices go offline.
- Improved scalability: It's easy to scale decentralized AI by increasing the number of edge nodes or devices, resulting in smoother and better data transmission.
Cons
- Trust deficit: Ensuring the quality and dependability of data on decentralized networks is crucial, as DAI algorithms require access to large datasets for accurate decision-making and predictions
- Not yet user-friendly: DAI systems present challenges due to their complex nature.
Should AI Be Decentralized?
Artificial intelligence is one of the most potent and expressive technological advancements we can encounter today, with its ability to recognize patterns, analyze data, and make decisions faster than human beings.
However, many of today's AI systems are centralized and owned by a few organizations.
Concentrating control can negatively impact AI's potential benefits, granting excessive influence to a few unchecked entities.
The giant corporations running AI systems own massive data stacks with costly data science teams that can easily create a cycle where their models generate more data, enriching their data sets and fortifying their dominance in the AI arena.
By relying on a single authoritative source, it's also easy for existing AI systems to generate biased results based on incomplete knowledge.
Moreover, centralized AI systems may use user data without transparency, raising concerns over data usage. Conversely, DAI distributes control of this powerful technology instead of concentrating its vast power, thereby mitigating the potential to hoard influence by a single entity.
As artificial intelligence advances, it becomes critical to decentralize its development and applications to create a trustless, permissionless system. Traditional open-source models may have some positive elements, but their undoing comes from the fact that they are built in silos instead of collaboratively.
To overcome the challenge caused by data silos, there's a need for open-source developers to coordinate and develop machine learning models with the ability to collaborate with and learn from each other. This collaborative approach is key to developing effective decentralized AI systems.
Advancements in blockchain technology and cryptography that can address the shortcomings of traditional AI models are now emerging. Distributing the development and governance of AI's power across different entities will enable artificial intelligence to progress better and provide checks and balances against possible manipulation by corporations or governments.
While centralized control could allow biased AI utilization against the interest of the masses, decentralized AI limits the possibility of an entity imposing prejudiced goals, incentives, or constraints.
FAQs
What is the decentralization of AI?
Decentralized artificial intelligence is an approach to AI that leverages blockchain technology in distributing, processing, and storing data across a network of devices (nodes). The system ensures that models and data are distributed across multiple devices from a centralized location.
Decentralized AI helps improve fairness and transparency, making it difficult for fraudsters to access and exploit data.
What issues does decentralized AI target?
Single entities control traditional centralized AI and can easily lead to tampering and manipulation of data. Decentralized AI addresses such challenges by preventing data hoarding so no organization can impose discriminatory constraints, goals, or incentives. This is crucial for such technology as it makes it inclusive and less prone to bias, besides eliminating unfairness and potential discrimination.
What is decentralized machine learning?
Decentralized machine learning (DML), or collaborative learning, distributes data across numerous network devices instead of centralizing it in one location. The learning technique trains algorithms across several decentralized servers or edge devices holding local data samples without switching them.